Table of content
The development of a gigantic data plan is undoubtedly not a piece of cake, and if you strive to remain relevant in the market, you need to have it ASAP. Data science integration helps businesses to obtain industry information on various aspects that is updated, optimized, and valuable. We will be discussing the top 5 data science integration challenges in your existing system in this article.[lwptoc title="5 Data Science Integration Challenges in Your Existing System" skipHeadingLevel="h1,h4,h5,h6"]Over the past decades, firms have gathered enormous data, and the number only increases with time. This immense quantity of data must be handled systematically to enable businesses to obtain previously undisclosed actionable insights. Such companies need a robust data strategy, and data science integration is an extremely crucial element.
Data Science Integration Challenges
Data integration is typically carried out in a data warehouse that involves advanced tools for hosting and extracting vast databases, assemblage, and a coherent display of information. You will have to tackle numerous obstacles in the process, even if you are using the latest tools.
Redundant and Obsolete Data
You will have incomplete, redundant, obsolete, and duplicate data if your company handles the data manually or lacks decent data entry standards. The same data could be inserted into various databases by multiple departments, thereby creating duplicates.Manual data updates may result in data entry errors or the inability to retain massive volumes of data. Even if you are not handling your data manually but not organizing your database regularly, you can also get in trouble. Consequently, your data will be inconsistent and unreliable and will result in a flawed analysis.The following graph represents how updated, and correctly handled data helps your company:
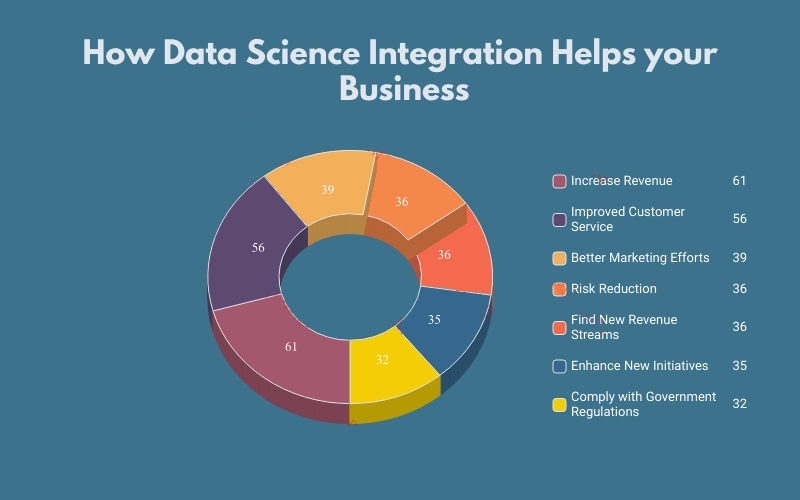
US Data 2017
Discrete Source System Technologies
Companies have to tackle the increase in the diversity of the data itself and the increasing variety of database technology behind it. This may involve a mixture of SQL, NoSQL, and data systems used in the cloud and the premises.
This issue is sorted using specially designed databases and the emergence of microservice architectures that split up databases into smaller parts and allocate them across business structures. While converting to a smaller architecture simplifies the creation of an application, further complicating the data science integration.A good company should catalog all of its existing data and adopt best practices to tackle the modern complex data landscape.
Accessing and Cleansing the Data
The data needs to be accessed in the proper format to pick the right data for analysis. It is challenging and time-consuming to grant permission from multiple organizations for the access of data.Data scientists can handle the data management system and other methods with the help of tools, like Stream, a software for the filtering of data and integration. The software enables all external data sources to be linked and aligned with the right layout.The following figure shows the options of Azure Stream Analytics:
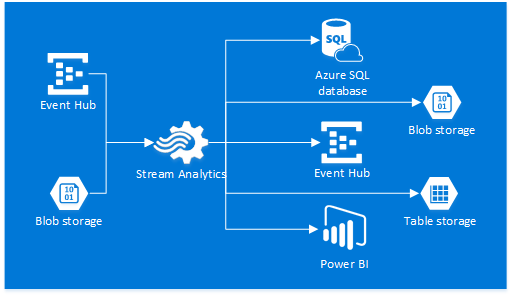
Image Source: Blend Master
Huge data quantities are difficult to manage, and every data scientist wishes to abstain from the databases full of contradictions and irregularities as corrupted data leads to incorrect results. To improve their overall precision and formatting, data scientists use data governance tools. Inaccurate and uncleaned data can result in a disaster for the company.
Scalability Challenge
The vast input of data from multiple sources into a single system allows data integration projects to evolve exponentially. Many companies understand that the need for more storage and power will quickly rise.Before choosing an integration solution, enterprises must predict the scale of the big data environment. They can also take a step-by-step approach. Each database is presented independently, has its value analyzed, prioritized, and incorporated into the overall big data plan one after the other. Data can be separated into separate datasets, such as financial details, revenue, and customer data. These should be prioritized and integrated one by one to scale up the operation eventually.For any company, there will always be a need for more storage with time. Many companies prefer cloud-based and hybrid systems as they offer the scalability to satisfy increasing criteria for data.
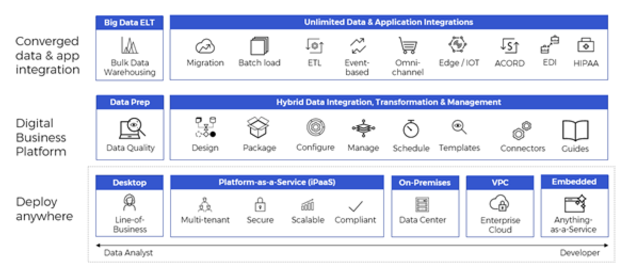
Image Source: Actian
The above figure shows the Actian DataConnect11 tool, which offers the possibility to design, manage, and handle integrations rapidly and efficiently in a robust, powerful hybrid integration solution in premises, cloud, and hybrid environments with no data types volumes constraints.
Integration Software
Is your company using an integration system? If yes, then still, you are not safe from the data science integration challenges. You don't have to pick an integration solution blindly, but you should opt for the RIGHT integration system. In some cases, you may have the right integration system, but you might be using it the wrong way, resulting in problems.
Conclusion
Data Scientists face at least three to four integration challenges annually in their existing systems. The most common obstacles are the shortage of data science expertise, corrupt and uncleaned data, and a lack of managerial support.A good company should be aware of the data integration challenges discussed above and properly tackle them. If any company has a sound design, it will efficiently collect and analyze the data and make the right data-driven decisions.